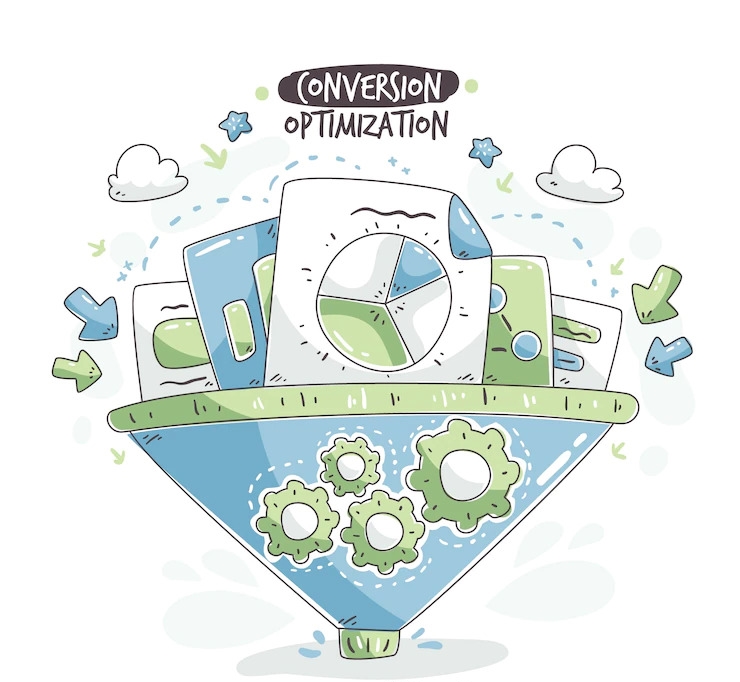
AI Down the Funnel: The Use Cases You’re Turning a Blind Eye to
As a prolongation of the BI revolution, the AI revolution compelled businesses to accumulate, speed up, consolidate, and deepen their data analytics, data science, and data handling skills. Companies are basing some of their key competencies around machine learning models as an organized method for making business choices as part of this revolution.
In finance, for example, the economic growth has resulted in an adequate supply of lending and investing capital due to low interest rates. The battle has shifted from “who will get sponsored” to “who will sponsor.” Lenders now must acquire market share swiftly and effectively, while simultaneously making sound judgments about possible risk/fraud, interest rates, and projected ROI. In the travel sector, machine learning models are used by online travel intermediaries and brokers to customise and match the best rates on hotels, and flights to clients in order to maximise sales while maintaining competitive pricing as per market standards.
Leveraging a company’s fundamental function around ML models has helped several organisations succeed in a variety of other domains, including insurance, marketing, and e-Commerce. Companies that utilise data to make automated verdicts, such as Ola, Airbnb, etc., have expanded enormously on this maxim. ML engines are now practically at the heart of every new technology-driven venture.
But there’s a catch! Data scientists are like a needle in a haystack, and developing the correct treasure trove of models to run a whole organisation is a difficult, monotonous task that necessitates practically all of the data science resources available to a corporation.
As these businesses expand and their basic business models and procedures become more stable, training models and controlling their life cycle in production become more efficient. As a result, they naturally begin to search for ways to improve other aspects of their organisation. When this is combined with the evident ROI predictive models deliver, the hunger for constructing more models across a company’s value chain soars.
Why aren’t businesses implementing machine learning across the board?
Companies do not expand their machine learning skills for a variety of reasons. They may be divided into three major types in general.
- The apparent resource shortfall: You believe that your data science resources should be dedicated to the core operations rather than supporting extra roles. Today’s AutoML tools, libraries, and capabilities, on the other hand, allow you to access fresh data and generate new models lot quicker than before.
- The organizational disparity: In many organisations, data science is either dispersed throughout the organisation or confined to a single function. What was formerly a risk team is now a possible consolidated data science team. When you begin to look at this team in a new light, you open your firm up to an infinite number of models.
- The dilemma of the perfectionist: Any auxiliary models you construct do not need to have the same high-quality metrics as your main models. There is opportunity for negotiation. It can still be a swift victory if a model’s output test is regularly superior than your existing random/rule-based solution.
Where can I make use of ML Models?
Top of the funnel – The traditional conjecture
Leads are costly in today’s highly competitive market. There are several approaches to maximise your spend, whether they are natural or sponsored. For example:
Outbound operations: Time and effort for outbound operations may be prioritised and optimised at the top of the funnel. Outbound prioritising examples include:
- Direct mail marketing is the process of prioritising which firms to spend money on in order to deliver physical mail.
- Messaging optimization entails giving your communications and targeted initiatives a personalised touch.
Promotional marketing: Getting the proper leads into your funnel is difficult; businesses are increasingly investing more time, money, and effort in deploying ML models for lead generation so that their internet and social media advertising expenditure results in greater conversion rates.
Top of the funnel models are especially effective in the financial sector since they provide a more targeted and less governed technique for avoiding spending marketing resources on riskier undertakings.
Moving down the funnel, take advantage of every drop of information
Every stage in the sales pipeline needs time and effort, whether it is contacting the customer, attending meetings, producing content, or performing any other physical labour.
As you go down the funnel, you lose leads, but the leads that sustain collect more and more data, which can enrich your model. So, why not make advantage of this information?
ML models can still be used to prioritise and concentrate the sales force on the relevant possibilities, especially when open opportunities outnumber available resources. Creating a model that assigns a real likelihood of closing (at all times) can assist your sales staff in properly prioritising their interactions.
The opposite side of the coin – stir up projections, accomplishment, and customer satisfaction
Putting together a customer success team is difficult. On the one hand, you want to ensure that you have a viable ratio of team members to clients, while also reducing churn and increasing the value of each individual customer.
Metric-led CS teams are unavoidable. There are several options available today to assist you track your customer interactions, involvement, clicks, and phone calls. These solutions assist you in gathering product feedback and even developing a few rules of thumb for consumers that leave. These guidelines were valid before the advent of business intelligence, but developing a churn prediction model that incorporates all of the data you’ve acquired about the client as well as extra enrichments can help your CS team prioritise their efforts and detect “flight risks” ahead of time. Why sit tight and wait for a client to begin the churning process when you can avoid it?
Consider going full-funnel
It has never been simpler to create and execute ML models. A model may be constructed everywhere there is data. Service desks, HR capacity estimates, and other minor but critical phases in the funnel
Machine learning portals, new libraries, and automated data mining and feature engineering tools enable data scientists to quickly develop, refine, and execute models. The use of the appropriate collection of skills and platforms maximises the productivity of your data science resources. Weeks of pre-processing, feature engineering, data selection, and model testing are no longer required. It’s a straightforward answer to a straightforward problem.
Your foundational models will continue to consume the majority of your time. A prudent risk model is not a luxury for an internet lending firm; it is a must. Any increase in the AUC might have a significant impact on the main business. However, if you haven’t been focusing on the many more machine learning use cases in your funnel, you are just operating at a loss, or worse, dumping money away.